Creation of composite marker from a stone
Contents
1.2. Creation of composite marker from a stone¶
1.2.2. Method¶
1.2.2.1. Module importations¶
import os
import json
import time
import wget
import tarfile
import numpy as np
from cv2 import aruco
import gbu # <- python -m pip install git+https://github.com/elmokulc/GBU_pose_classifier.git
import matplotlib.pyplot as plt
1.2.2.2. Import image dataset¶
You can download the images source from here
tar_filename = "GBU_pose_classifier-0.0.tar.gz"
if not os.path.isfile("./" + tar_filename):
url = "https://github.com/elmokulc/GBU_pose_classifier/archive/refs/tags/0.0.tar.gz"
filename = wget.download(url)
print("Download done !")
if not os.path.isdir("./_dataset"):
print("Files extraction...")
tar = tarfile.open(tar_filename, "r:gz")
tar.extractall()
tar.close()
!mkdir _dataset
! cp GBU_pose_classifier-0.0/dataset_subsample/* ./_dataset/
!rm -r GBU_pose_classifier-0.0
print("Importation done !")
Download done !
Files extraction...
Importation done !
1.2.2.3. Setting up dataset¶
# SETUP
image_directory = "./_dataset/"
metadata = json.load(open(image_directory + "metadata.json"))
camera_matrix = np.array(metadata["camera_matrix"])
distortion_coefficients = np.array(metadata["distortion_coefficients"])
md = float(metadata["aruco_marker_size"].split()[0])
marker_dimension = {i: md for i in range(1, 250)}
marker_size = 12.4079e-3
marker_dimension = {i: marker_size for i in range(1, 250)}
# Aruco settings
parameters = aruco.DetectorParameters_create()
parameters.cornerRefinementMethod = 3
parameters.cornerRefinementWinSize = 5
parameters.cornerRefinementMaxIterations = 100
1.2.2.3.1. Individual marker pose estimation¶
# STEP 1: PREPROCESSING
print("# BATCH INTITIALIZATION")
batch = gbu.calibration.ImageBatchCalibration(
aruco_dict=aruco.DICT_6X6_250,
parameters=parameters,
marker_dimension=marker_dimension,
output_directory="./_outputs_calibration/",
camera_matrix=camera_matrix,
distortion_coefficients=distortion_coefficients,
)
batch.load_image_batch(directory=image_directory)
batch.detect_markers(
plot_markers=False, enforce=False, meta_filename="metadata.p", protocol=4
)
_ = batch.estimate_pose()
# BATCH INTITIALIZATION
Dataframe export in Pickle
1.2.2.3.2. Graph theory & pre-optmization educated guess¶
data, graph, central_mk, cycles = batch.get_graph_data(
atomic_cycle=False, enabled_central_mk=True
)
gbu.utils.plot_graph(graph, title="Initial graph")
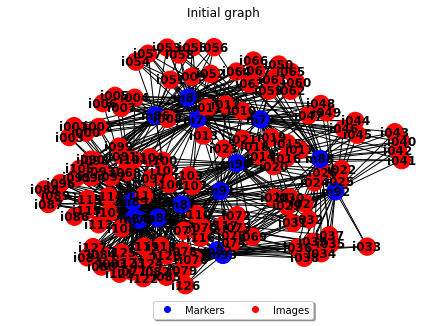
batch.get_good_class(
criterion=0.1,
alpha_criterion=1.5,
)
batch.graph_calibration()
full data = 1522 poses
no valid pose = 4 poses
out of cycle = 0 poses
good data = 461 poses
bad data = 1013 poses
ambigus data = 44 poses
full data = 505 poses
no valid pose = 0 poses
out of cycle = 0 poses
good data = 463 poses
bad data = 18 poses
ambigus data = 8 poses
full data = 471 poses
no valid pose = 0 poses
out of cycle = 0 poses
good data = 462 poses
bad data = 4 poses
ambigus data = 0 poses
(<Gbu Composite Marker w. 15 markers>, <Gbu Pose batch w. 110 entries>)
batch.plot_graph(title="Graph after cycle analysis")
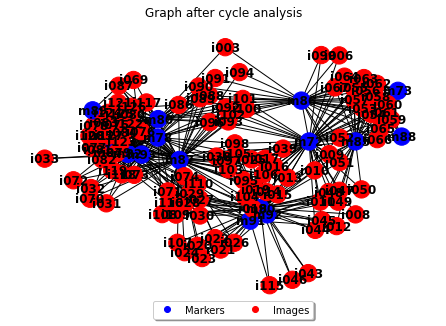
1.2.2.3.3. Least-square optimization¶
t0 = time.time()
compo, poseBatch, sol = batch.optimize_calibration()
t1 = time.time()
print(
"=> Ran optimization in {0}s for {1} poses".format(
(t1 - t0), len(batch.data_good_core)
)
)
=> Ran optimization in 33.598124742507935s for 462 poses
1.2.2.3.4. Reprojections error¶
batch.plot_reprojection_errors(
global_plot=True, individual_plots=False, plot_type="interactive"
)
import plotly.express as px
import plotly.graph_objects as go
data_projected_points = batch.projected_points()